Adaptive learning and personalization are gaining in popularity, and many ed tech startups offer products that claim to be adaptive. However, the field struggles with clear definitions of the terms adaptivity and personalization, and the science behind them is sometimes shaky. My goal with this post is to bring some structure into this conversation.
Adaptive and Personalized Learning
What exactly does Adaptive Learning mean? Often this term is used interchangeably with Personalized Learning, though some people (such as my friend Andrew Smith Lewis) use Personalization to describe approaches that go beyond Adaptivity. I may dive into the differences of these two constructs in one of my next posts. For now, I use the following definition: Adaptivity is an approach to the design of a learning system in which each learner is provided with the kind of experience he or she needs at any given time in order to be successful in reaching the intended (learning) outcomes.
Let’s take as example Lajune, biology major at a community college, who is taking Intro to Statistics. She had taken a stats course in high school and feels comfortable with this topic but is not all that interested. Her classmate Josh, majoring in communications, attended a high school where statistics wasn’t offered. He has less prior knowledge but is excited to learn. The Statistics instructor is using an adaptive platform for this class that incorporates video and stats simulations.
Let’s look at some of the questions this scenario raises.
What to adapt for?
One of the most interesting questions related to adaptivity is what to adapt for. If adaptivity means learners get what they need to succeed, then what exactly is the need are we talking about? Usually the focus is on cognitive variables – the learner’s current knowledge. But there are many other variables we could adapt for – the learners’ emotional state, their cultural background, or social variables, see Table 1 for examples. Clearly, current adaptive systems barely scratch the surface as far as the variable they adapt for.
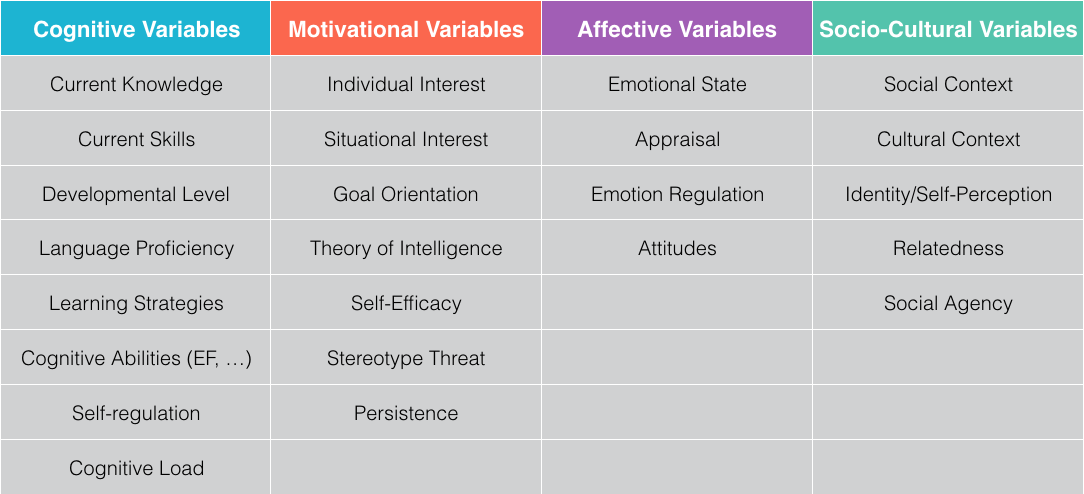
In our example, obvious candidates for adaptivity are the learners’ prior knowledge and level of motivation. But as the table shows, other variables could be considered, such learners’ executive functions (which has been linked to simulation exploration) or emotion experienced during learning, which have also shown to impact learning?
How do measure key variables for adaptation?
The process of adaptivity looks deceivingly simple at first glance. Once we have settled on one or more variables to adapt for, we need to determine a way to measure these variables while the learner is interacting with the system. This could be done through observation of the success in solving problems (cognitive variable), the amount of cognitive load they experience during learning (cognitive variable), the use of biometrics such as EDA, GSR, or HRV to diagnose experienced emotions (affective variable), the use of location and proximity sensors to measure with whom people interact during learning (social variable), or through analysis of user logs to diagnose the student’s persistence or situational interest (motivational variable). Once this information is available, a learner model is updated. This model allows us to predict specific learner states, based on which decisions have to be made as to what adaptive response is appropriate.
What needs to be considered before a variable is chosen as basis for an adaptive learning system, however, is (1) Is the variable relevant to achieve the intended learning outcomes? (2) Is there is enough learner variability on the variable to justify the need for adaptivity?, and (3) Is there is sufficient knowledge on how to adapt to learner differences along this variable? This last issue is critical as for many of the variables listed, the necessary research of how to adapt had not yet been conducted.
In our example, the simulations could be used to generate data that allows us to measure learners’ current knowledge. Motivation can be assessed through self-reports (e.g., a survey). A convenient way to measure emotion during a class is through repeated brief self-reports, despite the obvious shortcomings of this method.
How to adapt?
The question of how to respond to a specific diagnosed learner state does not have simple answers. In fact, despite decades of research on attribute-by-treatment interactions, our knowledge of what responses are useful is highly limited. Take the example of a frustrated learner. If our emotion detection determines that the learner is in a frustrated state, what is the appropriate response? Would you want to be told by a computer that you “seem frustrated”? Or would you appreciate the opportunity to change activities? Or, perhaps, should an animated empathetic agent model a more positive emotional state? In any case, the cycle of adaptivity is depicted in Figure 1. As a learner performs in a learning environment, the system diagnoses variables of interest that can be affective, cognitive, motivational, or socio-cultural. Based on this assessment, the system adaptively provides the learner with the kind of instruction he or she needs. But what exactly does this adaptivity look like? In order to sort out the many different ways of designing adaptive learning, I decided to begin developing a taxonomy.
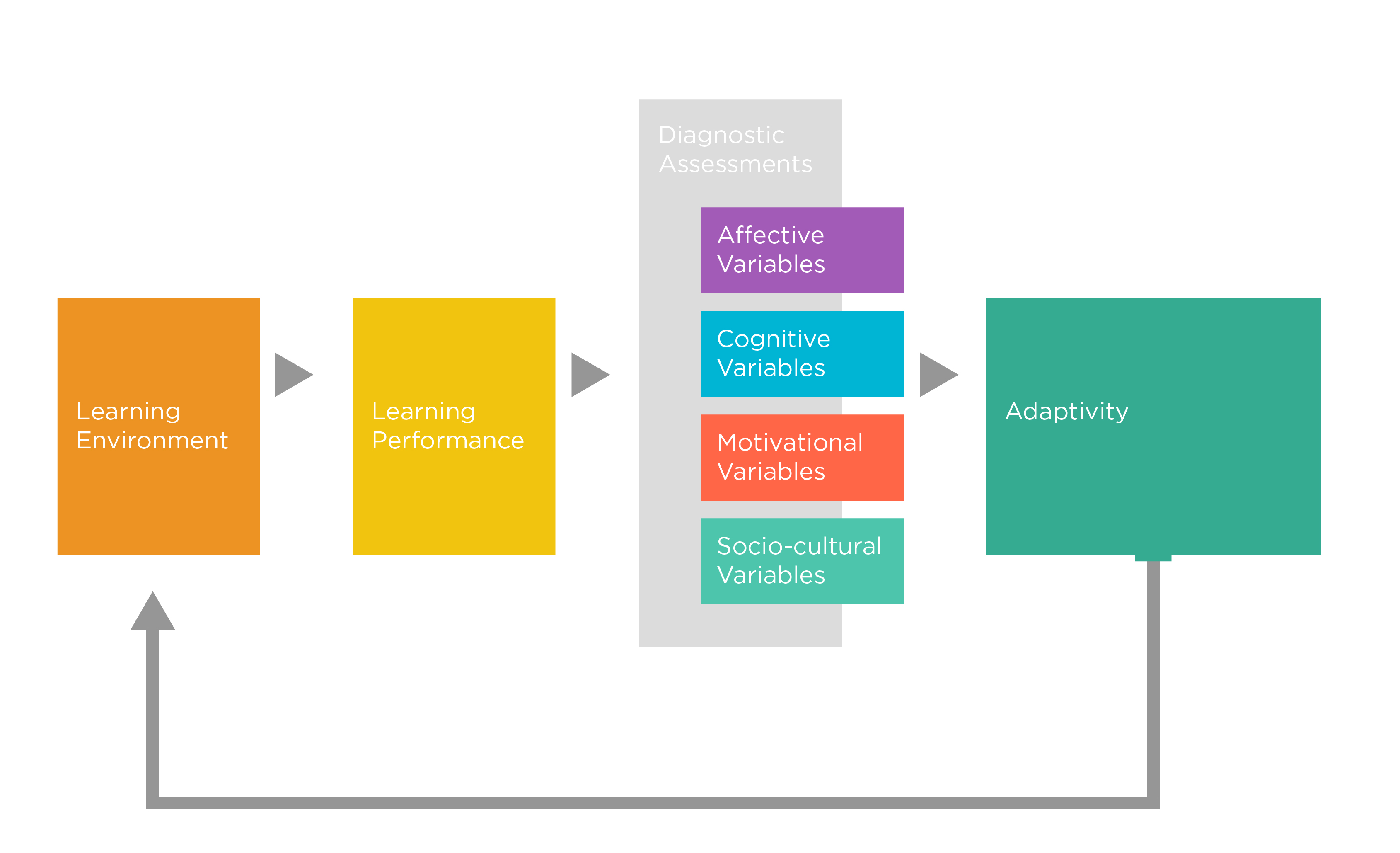
A Taxonomy of Adaptivity
Based on the learner model, there are several ways of designing adaptivity for learning. Principally, we can adapt in five different ways (see Figure 2):
- In preparation of learning
- As part of the core learning activity
- In the support learners are given
- During assessment of learning
- In the progression between courses
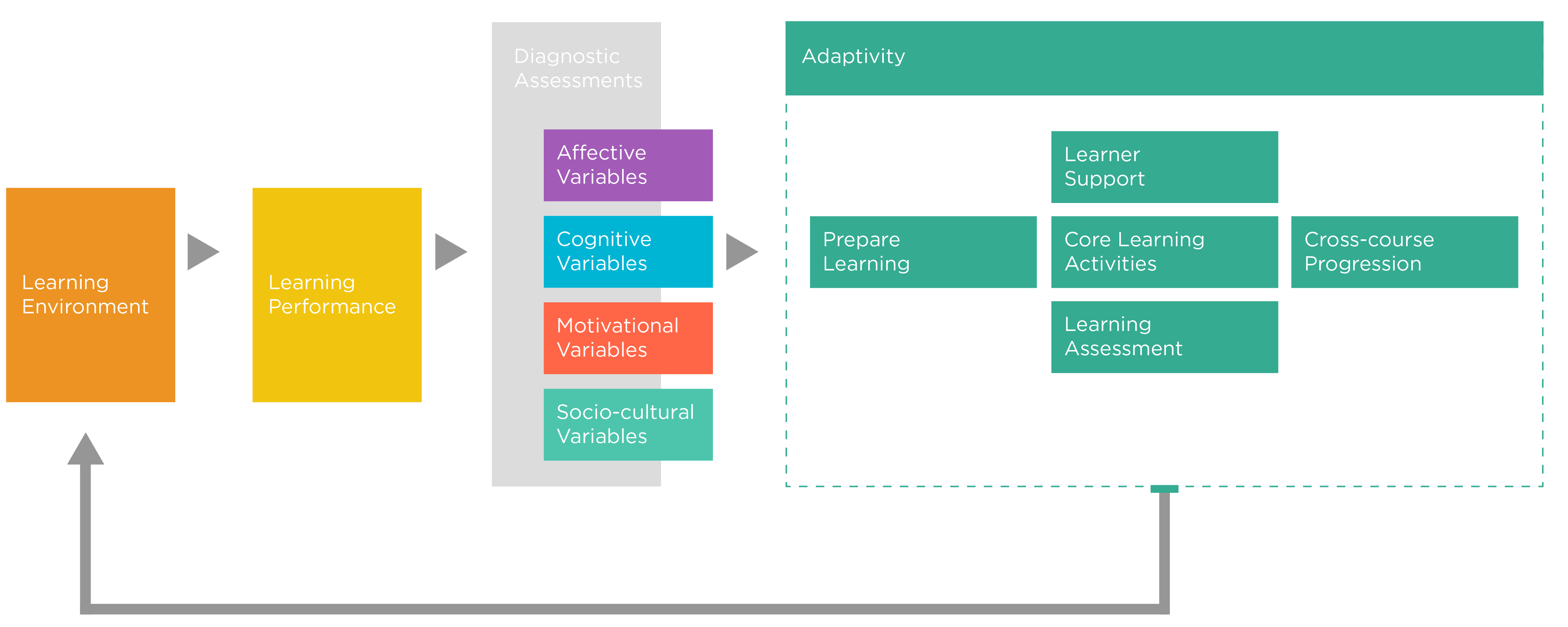
Core Learning Activity
The most involved form of adaptive learning concerns the core learning activity. Systems using this type of adaptivity make individual options available to learners that may involve the type of interactions they are provided, the mode of representation of information learners are given, the progression of difficulty as well as the conceptual progression they experience (Intelligent Tutoring Systems), the way rehearsals are scheduled (Spaced Repetition Systems), and the scaffolds, cues, feedback and guidance learners receive.
Learner Support
Adaptive learner support involves resources and guidance that become available outside of, but related to the core learning activity. For example, after completing a particular unit, a learner may be given individual feedback in the form of a dashboard that describe areas of strength and weakness, and be pointed to targeted resources and guidance, potentially in different modes.
Preparation of Learning
There are a number of conditions which do not call for an adaptive core learning activity or adaptive learner support. For example, a student not interested in the subject matter or lacking sufficient language proficiency may be better served by adaptive pre-learning interventions, such as a motivational intervention or language training. Similarly, adaptive preparation of learning may include remedial learning and learning strategies training. Also popular is cognitive skills training, such as training of executive functions, in preparation of future learning.
Learning Assessment
Assessment has a long history of using adaptivity, namely in Computer Adaptive Testing. This method uses items with different levels of difficulty to adapt to the learners’ level of knowledge. However, there are other forms of adaptivity that can be used in assessment, especially related to the mode of test items and mode of responses, testing frequency, and the reporting mode of test results.
Cross-course Progression
Adaptivity can also take place on a macro-level, by making specific recommendations about the sequence of courses to take for an entire curriculum. Universities are adopting this method, especially for undergraduates, to optimize course sequences for their students. Additional adaptivity at this level could include decisions about pre- and co-requisites, electives, and even choice of majors and minors.
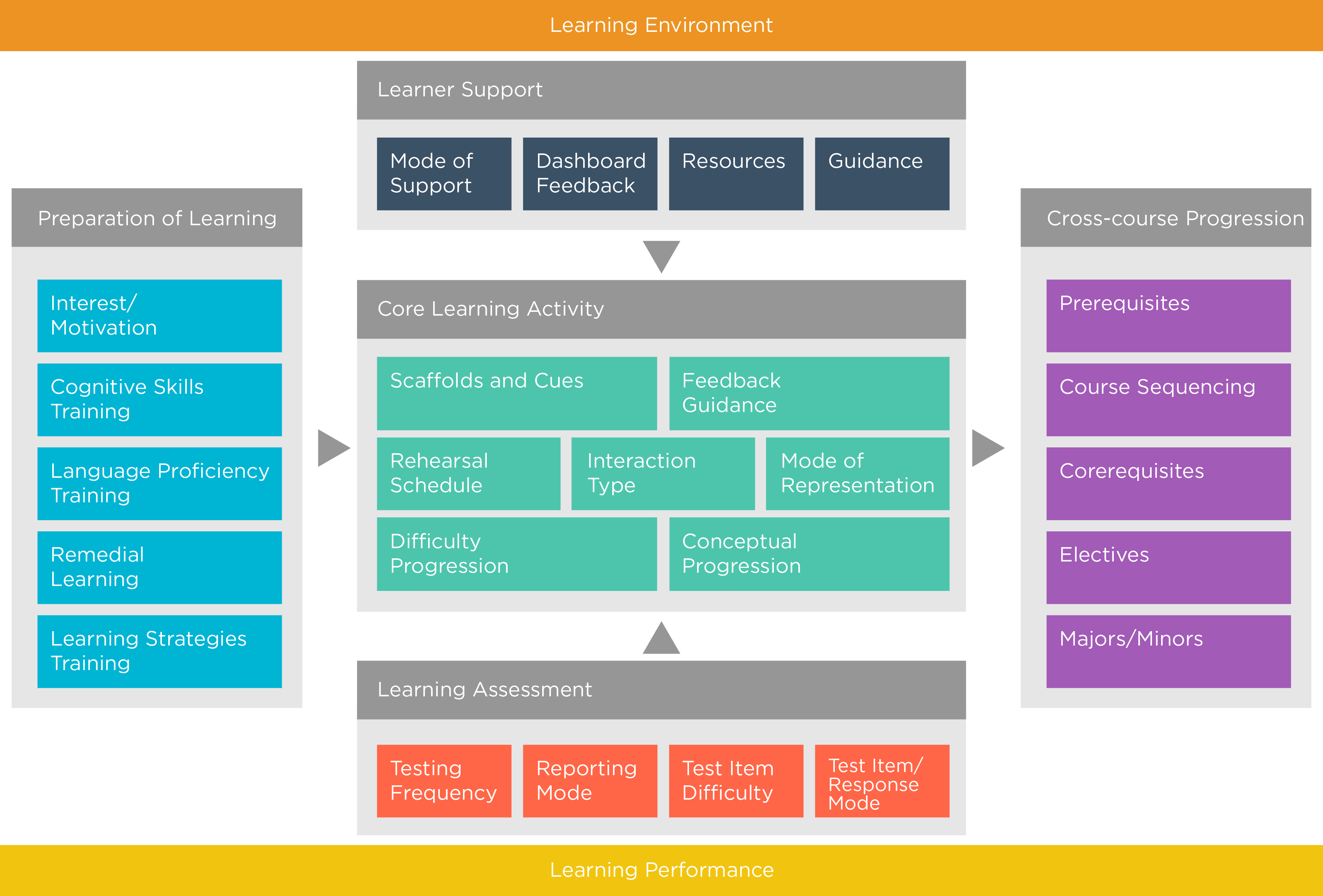
In our example, the adaptive response to different levels of knowledge could be to adjust the difficulty progression and the conceptual progression. In order to accommodate different levels of executive functions, there could be different scaffolds and different forms of representing key concepts as simulation or animation.
A Few Concluding Thoughts
It is important to note that this taxonomy of adaptive learning gives examples of different types of adaptive responses of a learning environment. I of course do not advocate using several or even all of these types; in fact, even implementing one of them in a learning system will pose significant challenges. I also believe that neither the list of variables that a system could diagnose and respond to (Table 1), nor the ways to respond (Figure 3) is exhaustive. I invite readers to send additional variables or types of adaptive responses. If they fit my model, I’ll include them with the proper attribution.
Another important question to consider is how exactly an adaptive learning system implements any of the types of adaptivity in Figure 3. Educators and researchers alike stress the importance of learner’s self-regulation of learning and of their emotions, and an adaptive system should not eliminate the need for such self-regulation by managing the learner’s experience. Instead of making decisions for the learner based on diagnosed need, the system could make available better options for the learner to choose from.
Overall, adaptive learning environments have an incredible potential to optimize a learner’s individual learning experience and outcome. However, despite the many claims by vendors offering these systems, we are with few exceptions still at the beginning of an understanding of how to adapt learning for specific learner variables. This blog is therefore more of a research agenda than a description of what is already possible. My CREATE lab at NYU has been investigating several of these variables, and we will continue to do so in the future.